AI Applications for Cloud & Communications – A Five-Minute Overview
AI (Artificial Intelligence) already has a significant impact on the cloud and communications industry and will most likely revolutionize various aspects of sales, operations, customer service, network & infrastructure management in the upcoming years:
- According to McKinsey, AI applications could reduce network operations and maintenance costs by 25% to 35%,
- Juniper Research estimates that chatbots could save telecom operators $11 billion annually saving over 3.5 billion customer service hours,
- Accenture states that AI-driven personalization could increase telecom operators’ revenue by 20%,
- By Deloitte, predictive maintenance powered by AI can reduce maintenance costs by up to 40%.
AI is a hot topic and as such is surrounded by a lot of hype – this post is an attempt to bring clarity to the matter and highlight practical applications of AI for cloud and communications providers.
What is AI?
AI is intelligence exhibited by computer software by providing functions typically associated with human cognition (learning, reasoning, decision making, etc.).
From the practical perspective of applications for cloud & communications providers, AI can be classified as follows:
- Natural Language Processing (NLP) is the ability to understand, analyze and generate written and spoken human language. NLP applications include chatbots, virtual assistants, natural language data queries, calculating customer sentiment scores and others.
- Machine Learning is the ability to learn and improve over time, without being explicitly programmed. ML applications include fraud analysis, churn predictions, outage predictions and others.
- Generative AI is the ability to create brand new content (text, images, videos, computer code). ChatGPT is the most popular generative AI.
- Non-adaptive algorithms can mimic intelligent behavior, but must be programmed up-front, and unlike ML do not change their behavior over time. Non-adaptive algorithms include many traditional optimization techniques, network routing, traffic prioritization and others.
Other AI classifications exist (e.g., supervised vs. non-supervised, strong vs. weak), however they are not as important from a practical standpoint.
The following sections follow a typical Quote-to-Cash (QtC) process for cloud and communications services (sales order management & service delivery → support → upselling & cross-selling) and highlight potential AI applications at each stage.
AI in cloud & communications service sales
Opportunity scoring and prioritization. AI can be used to assess the likelihood of winning opportunities to concentrate sales efforts where they can yield best outcomes. The decision can be driven by multiple parameters such as product(s) sold, customer type, existing vs. new customer, current sales cycle stage and others.
Opportunity owner assignment. AI can determine who is the most likely salesperson to win a deal based on opportunity parameters (customer account, product(s) sold, product(s) offered, etc.) and person’s prior win history and (re-)assign the opportunity accordingly.
Optimum vendor selection. For re-sold products, the best vendors can be selected automatically based on past delivery experience, number of vendor issues, ticket TTRs and other factors.
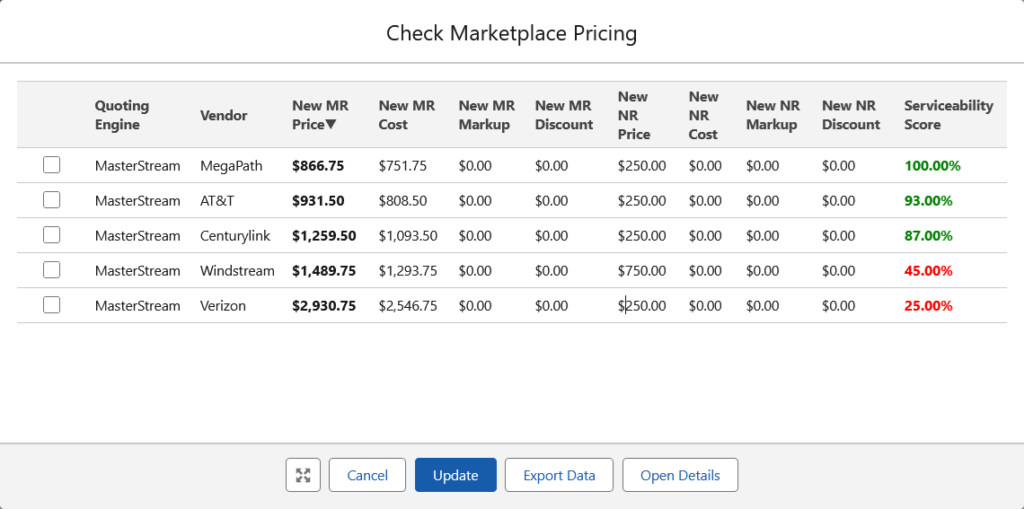
Additional product suggestions. Algorithms can suggest additional products complementing current purchases. e.g. customers buying a server will most likely buy additional storage, customers buying SD-WAN will most likely buy a SD-Security add-on, etc.
Upselling based on usage intelligence. AI can discover potential upgrade opportunities (e.g., more storage or bandwidth, different products, etc.) for easy upselling based on usage and performance metrics for networks, computers and software.
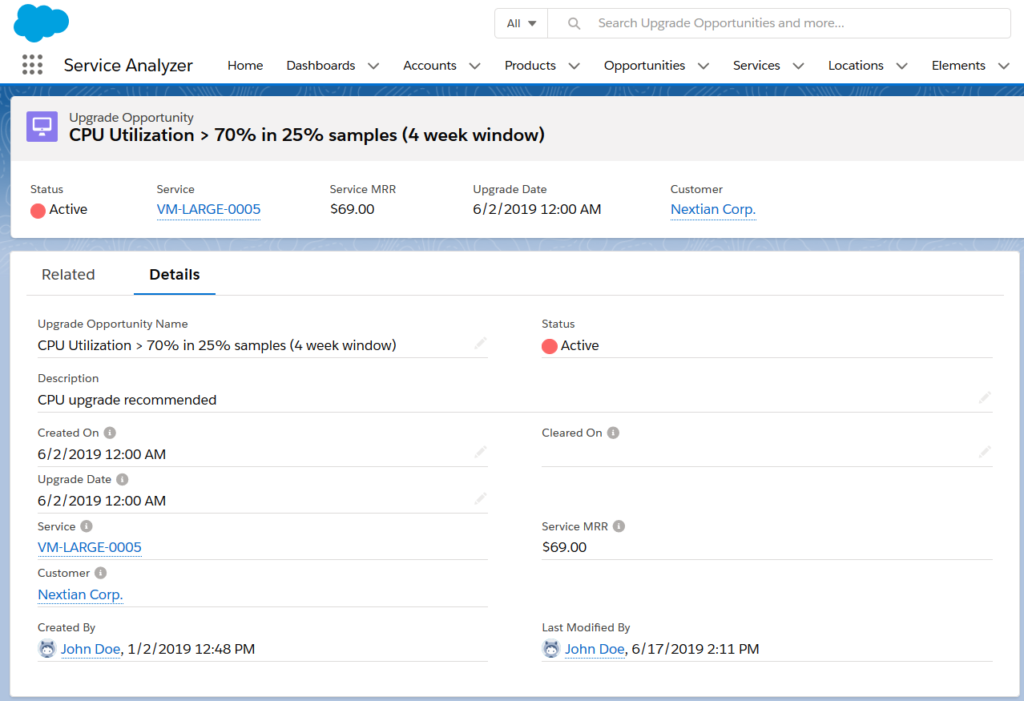
AI in order management and service delivery
Order & task routing. Algorithms can make order and task assignment decisions to drive delivery efficiency. Tasks assignments can be made based on type of product delivered, experience with a particular customer, employee skills and others.
Order sentiment analysis. Similarly to sentiment scores for cases, order sentiment scores indicate positive, negative, or neutral (typically represented as 1, -1 and 0) customer experience with service delivery. Order sentiment score can be calculated based on order comments made by a customer during delivery process.
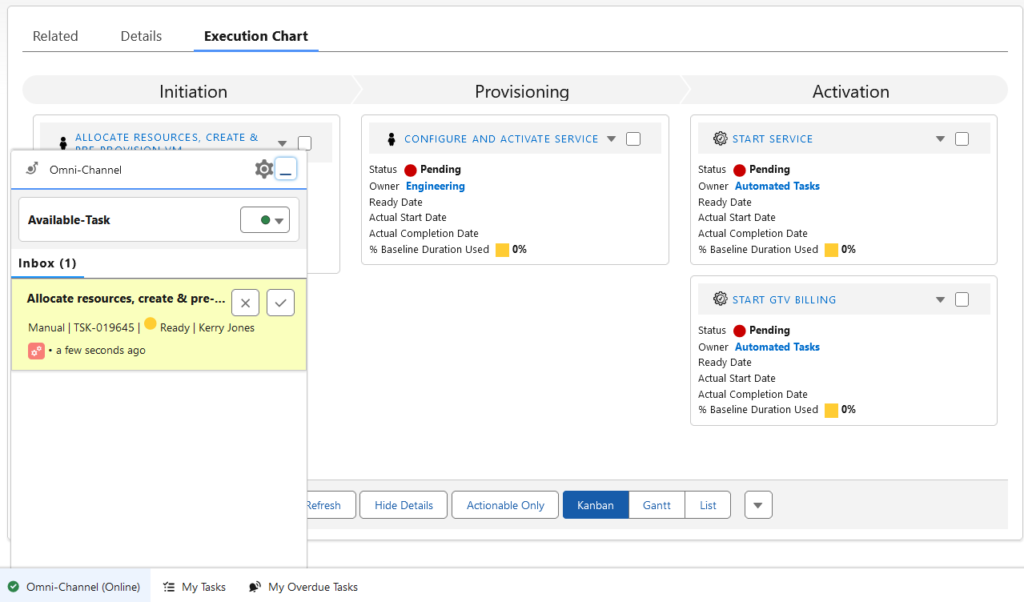
AI in infrastructure management and service monitoring
Service monitoring and fault diagnostics. For complex services involving multiple elements (servers, storage, power, network connections, routers, etc.), AI can speed up fault diagnostics by cross-referencing and aggregating information about statuses of individual service elements, reducing issue resolution times.
Predictive outage and anomaly detection. AI can learn baseline usages of services and detect anomalies (such as possible fraudulent usage) and predict outages (the server temperature goes up over time without going down, I/O errors or slower read times indicating future disk problems and others) leading to reduced downtimes and higher customer satisfaction.
Automated infrastructure supervision. Algorithms can prioritize network traffic, manage load-balancing and route requests, helping providers maximize their resource utilization and service performance, reducing operating costs.
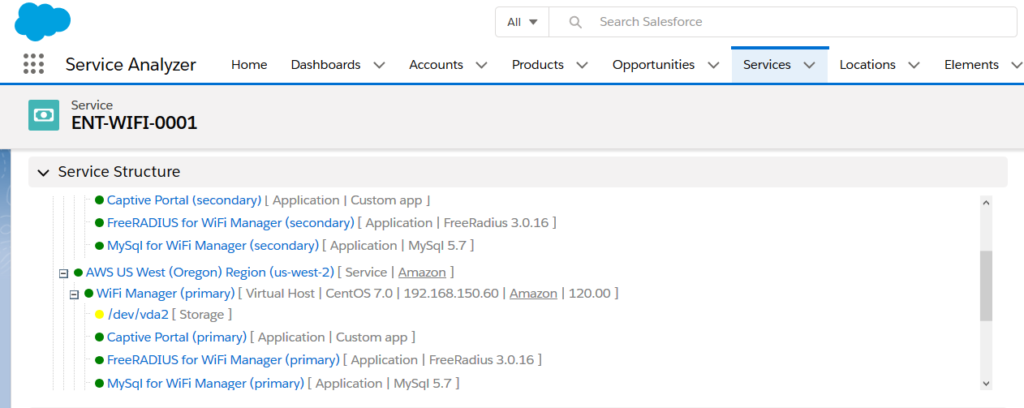
AI in support / ticketing
Chatbots are the first thought when it comes to AI in customer support, however they have one significant disadvantage: customers don’t like them (just like they don’t like IVRs), and usually choose ‘talk to a human’ option. For higher value, complex services a technician/engineer usually has to be involved, however AI can still be of great help with:
Case routing. Algorithms can route issues to the most qualified technicians depending on problem type, product, required skills and others, shortening TTRs and enabling providers to use resources effectively.
Root cause analysis. Automated root-cause analysis (even when not 100% accurate), can help technicians shorten overall issue resolution time, especially for complex services involving multiple elements of various types such as servers, routers, storage, Internet connections and others.
Resolution suggestions. Based on ticket description, product and infrastructure status AI can be used to suggest potential problem remedies.
Response generation. AI can be used to generate support responses that can be modified by support personnel, shortening times required for communications. This is similar to using traditional response templates but enabling more customized message content based on the case information.
Sentiment analysis. A sentiment score measures the emotional tone expressed by the customer in ticket description and subsequent comments. The sentiment score typically indicates whether the ticket expresses positive, negative, or neutral (typically valued as 1, -1 and 0) experience with the product or service. Sentiment score is a complementary measure to CSAT but a) can be measured for all tickets while surveys are rarely answered, and b) can be obtained much earlier in the process.

Security threat and fraud detection with AI
AI is great at analyzing large data sets and looking for patterns, and as such is well suited to detect security threats and fraud. Common techniques used for threat and fraud detection include:
Behavioral analysis. AI can establish baseline behavior patterns and use them to detect abnormal / suspicious user activities that may suggest fraudulent or unauthorized actions.
Usage analysis. AI can establish baseline usage metrics and apply them to flag anomalies that may indicate intrusions, malicious activities, Distributed Denial of Service (DDoS) attacks, unauthorized data copying or malware infections.
Threat intelligence. AI is well suited to detect evolving threats such as spam, phishing attempts and malware. ML models enable recognition of malicious communication by detecting illegitimate language and formatting patterns. They also can detect and predict new malware types based on deviations from normal file patterns.
While all the above can be achieved by traditional, non-adaptive algorithms (e.g., ones employed in firewalls), AI can give better results in the long term due to its ability to learn.
AI in reporting & analytics
Natural Language BI (a.k.a. Natural Language Reporting or Querying) enables report generation by asking questions in plain English without knowledge of SQL, making self-analytics available to non-technical users. It can also provide contextual explanations as well as pattern, trend and anomaly insights to help users better understand the data and improve decision making.
Summary
AI (Artificial Intelligence) already has and will continue to impact the cloud and communications industry across the entire Quote-to-Cash spectrum including sales, operations, customer service, network & infrastructure management.
AI adoption will be driven by the following factors:
- Reduction in network operations and maintenance costs,
- Efficiency in customer service (fewer hours spent on issue resolution),
- Personalization of communication for improved user experience,
- Reduction of costs with predictive maintenance,
- Improved threat and security protection.
About Nextian
Nextian offers software products and services helping cloud & communications providers improve their Quote-to-Cash to drive revenue growth and customer base retention.
Contact us today to find out how we can help your business grow!
- McKinsey: Smartening up with Artificial Intelligence (AI)
- Juniper Research: Chatbots to Deliver $11bn in Annual Cost Savings
- Accenture: Using AI and data to ignite profitable revenue growth for high-tech companies
- Deloitte: Predictive Maintenance – Taking pro-active measures based on advanced data analytics to predict and avoid machine failure